Category Archives: KB
- 08/03/2021
-
Enter your password to view comments.
- 07/03/2021
-
Enter your password to view comments.
Protected: AI框架搭建顺序
Category : Blockchain and AI python
- 25/02/2021
-
Enter your password to view comments.
- 22/02/2021
-
0
一文读懂Supervised vs Unsupervised Learning: Key Differences
Category : AI Blockchain and AI Sinovation Startup Workshop
What is Supervised Machine Learning?
In Supervised learning, you train the machine using data which is well “labeled.” It means some data is already tagged with the correct answer. It can be compared to learning which takes place in the presence of a supervisor or a teacher.
A supervised learning algorithm learns from labeled training data, helps you to predict outcomes for unforeseen data. Successfully building, scaling, and deploying accurate supervised machine learning Data science model takes time and technical expertise from a team of highly skilled data scientists. Moreover, Data scientist must rebuild models to make sure the insights given remains true until its data changes.
In this tutorial, you will learn
- What is Supervised Machine Learning?
- What is Unsupervised Learning?
- Why Supervised Learning?
- Why Unsupervised Learning?
- How Supervised Learning works?
- How Unsupervised Learning works?
- Types of Supervised Machine Learning Techniques
- Types of Unsupervised Machine Learning Techniques
- Supervised vs. Unsupervised Learning
What is Unsupervised Learning?
Unsupervised learning is a machine learning technique, where you do not need to supervise the model. Instead, you need to allow the model to work on its own to discover information. It mainly deals with the unlabelled data.
Unsupervised learning algorithms allow you to perform more complex processing tasks compared to supervised learning. Although, unsupervised learning can be more unpredictable compared with other natural learning deep learning and reinforcement learning methods.
Why Supervised Learning?
- Supervised learning allows you to collect data or produce a data output from the previous experience.
- Helps you to optimize performance criteria using experience
- Supervised machine learning helps you to solve various types of real-world computation problems.
Why Unsupervised Learning?
Here, are prime reasons for using Unsupervised Learning:
- Unsupervised machine learning finds all kind of unknown patterns in data.
- Unsupervised methods help you to find features which can be useful for categorization.
- It is taken place in real time, so all the input data to be analyzed and labeled in the presence of learners.
- It is easier to get unlabeled data from a computer than labeled data, which needs manual intervention.
How Supervised Learning works?
For example, you want to train a machine to help you predict how long it will take you to drive home from your workplace. Here, you start by creating a set of labeled data. This data includes
- Weather conditions
- Time of the day
- Holidays
All these details are your inputs. The output is the amount of time it took to drive back home on that specific day.
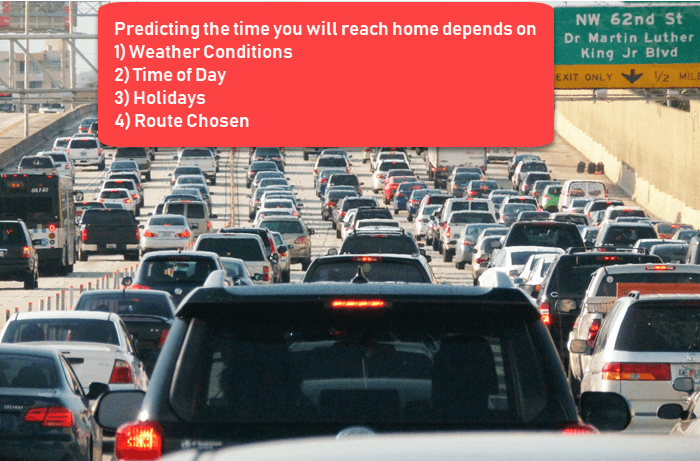
You instinctively know that if it’s raining outside, then it will take you longer to drive home. But the machine needs data and statistics.
Let’s see now how you can develop a supervised learning model of this example which help the user to determine the commute time. The first thing you requires to create is a training data set. This training set will contain the total commute time and corresponding factors like weather, time, etc. Based on this training set, your machine might see there’s a direct relationship between the amount of rain and time you will take to get home.
So, it ascertains that the more it rains, the longer you will be driving to get back to your home. It might also see the connection between the time you leave work and the time you’ll be on the road.
The closer you’re to 6 p.m. the longer time it takes for you to get home. Your machine may find some of the relationships with your labeled data.
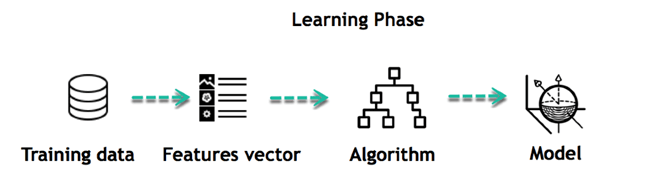
This is the start of your Data Model. It begins to impact how rain impacts the way people drive. It also starts to see that more people travel during a particular time of day.
How Unsupervised Learning works?
Let’s, take the case of a baby and her family dog.
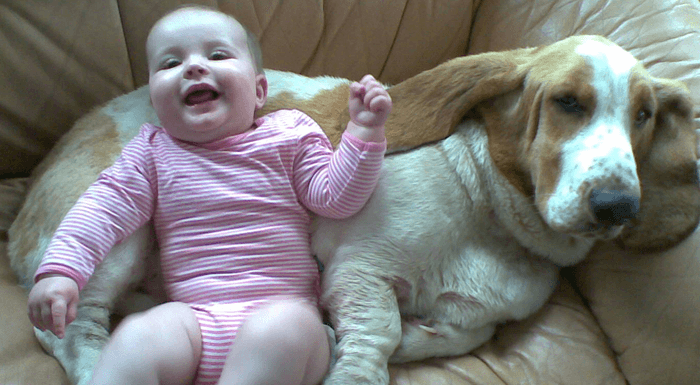
She knows and identifies this dog. A few weeks later a family friend brings along a dog and tries to play with the baby.
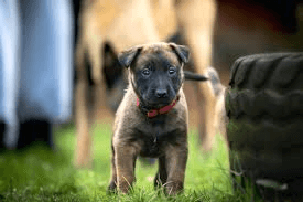
Baby has not seen this dog earlier. But it recognizes many features (2 ears, eyes, walking on 4 legs) are like her pet dog. She identifies a new animal like a dog. This is unsupervised learning, where you are not taught but you learn from the data (in this case data about a dog.) Had this been supervised learning, the family friend would have told the baby that it’s a dog.
Types of Supervised Machine Learning Techniques
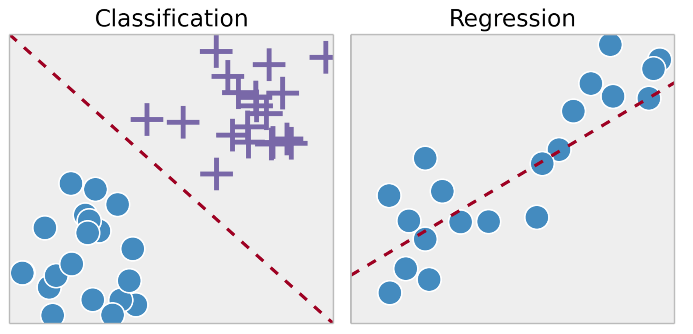
Regression:
Regression technique predicts a single output value using training data.
Example: You can use regression to predict the house price from training data. The input variables will be locality, size of a house, etc.
Classification:
Classification means to group the output inside a class. If the algorithm tries to label input into two distinct classes, it is called binary classification. Selecting between more than two classes is referred to as multiclass classification.
Example: Determining whether or not someone will be a defaulter of the loan.
Strengths: Outputs always have a probabilistic interpretation, and the algorithm can be regularized to avoid overfitting.
Weaknesses: Logistic regression may underperform when there are multiple or non-linear decision boundaries. This method is not flexible, so it does not capture more complex relationships.
Types of Unsupervised Machine Learning Techniques
Unsupervised learning problems further grouped into clustering and association problems.
Clustering
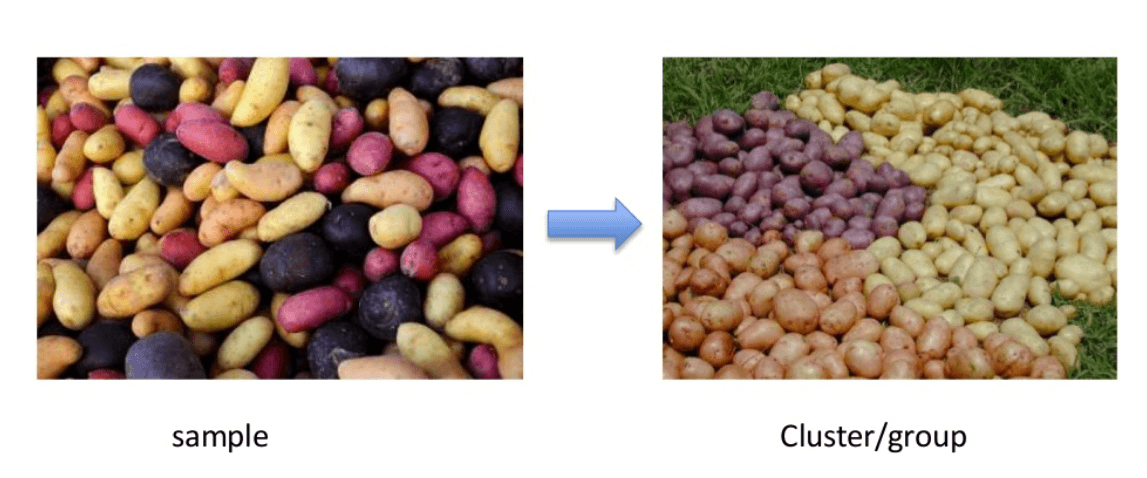
Clustering is an important concept when it comes to unsupervised learning. It mainly deals with finding a structure or pattern in a collection of uncategorized data. Clustering algorithms will process your data and find natural clusters(groups) if they exist in the data. You can also modify how many clusters your algorithms should identify. It allows you to adjust the granularity of these groups.
Association
Association rules allow you to establish associations amongst data objects inside large databases. This unsupervised technique is about discovering exciting relationships between variables in large databases. For example, people that buy a new home most likely to buy new furniture.
Other Examples:
- A subgroup of cancer patients grouped by their gene expression measurements
- Groups of shopper based on their browsing and purchasing histories
- Movie group by the rating given by movies viewers
Supervised vs. Unsupervised Learning
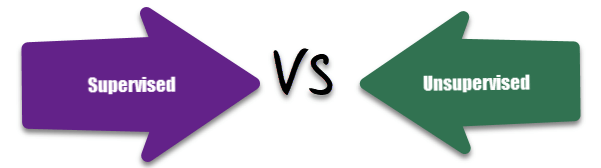
Parameters | Supervised machine learning technique | Unsupervised machine learning technique |
Process | In a supervised learning model, input and output variables will be given. | In unsupervised learning model, only input data will be given |
Input Data | Algorithms are trained using labeled data. | Algorithms are used against data which is not labeled |
Algorithms Used | Support vector machine, Neural network, Linear and logistics regression, random forest, and Classification trees. | Unsupervised algorithms can be divided into different categories: like Cluster algorithms, K-means, Hierarchical clustering, etc. |
Computational Complexity | Supervised learning is a simpler method. | Unsupervised learning is computationally complex |
Use of Data | Supervised learning model uses training data to learn a link between the input and the outputs. | Unsupervised learning does not use output data. |
Accuracy of Results | Highly accurate and trustworthy method. | Less accurate and trustworthy method. |
Real Time Learning | Learning method takes place offline. | Learning method takes place in real time. |
Number of Classes | Number of classes is known. | Number of classes is not known. |
Main Drawback | Classifying big data can be a real challenge in Supervised Learning. | You cannot get precise information regarding data sorting, and the output as data used in unsupervised learning is labeled and not known. |
Summary
- In Supervised learning, you train the machine using data which is well “labeled.”
- Unsupervised learning is a machine learning technique, where you do not need to supervise the model.
- Supervised learning allows you to collect data or produce a data output from the previous experience.
- Unsupervised machine learning helps you to finds all kind of unknown patterns in data.
- For example, you will able to determine the time taken to reach back come base on weather condition, Times of the day and holiday.
- For example, Baby can identify other dogs based on past supervised learning.
- Regression and Classification are two types of supervised machine learning techniques.
- Clustering and Association are two types of Unsupervised learning.
- In a supervised learning model, input and output variables will be given while with unsupervised learning model, only input data will be given
-
Enter your password to view comments.
Protected: 解决python中使用plot画图,图不显示的问题
Category : python
- 21/02/2021
-
Enter your password to view comments.
-
Enter your password to view comments.
Protected: DCGAN的创新
Category : AI Blockchain and AI
- 17/02/2021
-
Enter your password to view comments.
Protected: 啥也不说了,致敬
Category : Easi Design python
- 16/02/2021
-
0
python字典值默认为列表类型 defaultdict(list)
Category : python
from collections import defaultdict
index_dic = defaultdict(list)
创建的空的字典,默认了每个键值对中的值为空的列表
- 14/02/2021
-
0
Matplotlib Savefig() For Different Parameters in Python
Category : python
Contents of Tutorial
- Syntax of Matplotlib savefig()
- Parameters:
- Return type
- Example of Matplotlib Savefig() in Python
- Savefig() with other parameters
- Matplotlib Savefig() formats
- Change size using Matplotlib Savefig() in Python
- Conclusion
Syntax of Matplotlib savefig()
savefig(fname, dpi=None, facecolor=’w’, edgecolor=’w’, orientation=’portrait’, papertype=None, format=None, transparent=False, bbox_inches=None, pad_inches=0.1, frameon=None, metadata=None)
Parameters
- fname: (str or path-like or file-like) It determines the output format, and the file saves as fname. If the format is not present, then the format is the extension of fname, if there is one. If the fname has no extension, then the file is saved with default: ‘png,’ and the appropriate extension is appended to the fname.
- dpi: Resolution in dots per inch.
- facecolor(color or ‘auto’, default: ‘auto’): The facecolor of the figure. If ‘auto’, use the current figure facecolor.
- edgecolor(color or ‘auto’, default: ‘auto’): The edgecolor of the figure. If ‘auto’, use the current figure edgecolor.
- orientation – {‘landscape,’ ‘portrait’}: Currently only supported by the postscript backend.
- format(str): The file format, e.g. ‘png’, ‘pdf’, ‘svg’, … The behavior when this is unset is documented under fname.
- papertype: Papertypes can be “a0 to a10”, “executive,” “b0 to b10”, “letter,” “legal,” “ledger.”
- bbox_inches: Only a given portion of the figure is saved. Set it as “tight” for the proper fit of the saved figure.
- pad_inches: (default: 0.1) Amount padding around the saved figure.
- transparent: Makes the background of the picture transparent. The figure patch will also be transparent unless face color and/or edgecolor are specified via kwargs.
Return type
The matplotlib savefig() function saves the plotted figure in our local machines.
Example of Matplotlib Savefig() in Python
1 2 3 4 5 6 7 8 9 10 11 12 13 14 15 | import matplotlib.pyplot as plt # creating plotting data x_axis =[1, 4, 9, 16, 25, 36, 49, 64, 81, 100] y_axis =[1, 2, 3, 4, 5, 6, 7, 8, 9, 10] # plotting plt.plot(x_axis, y_axis) plt.x_label("X") plt.y_label("Y") # saving the file plt.savefig("squares.png") plt.show() |
OUTPUT:

EXPLANATION:
In the above example, a plot is drawn using the input values of the x_axis and y_axis. Once the plotting is over, it is first saved using the savefig() function. Only the ‘fname,’ given as “Squares.png,” is an argument to the matplotlib savefig() function. The plotted figure is thus saved with the file name Squares under .png format in the local system.
Savefig() with other parameters
1 2 3 4 5 6 7 8 9 10 11 12 13 14 15 16 | import matplotlib.pyplot as plt # creating data and plotting a histogram x =[1, 4, 9, 16, 25, 36, 49, 64, 81, 100] plt.hist(x) # saving the figure. plt.savefig("squares1.png", bbox_inches ="tight", pad_inches = 1, transparent = True, facecolor ="g", orientation ='landscape') plt.show() |
OUTPUT:

EXPLANATION:
Firstly, in the above example, a histogram is plotted by importing the Matplotlib library using the various input values. The figure is saved in the local system using the Matplotlib savefig() in Python. Parameters as arguments are necessary to obtain the saved figure as desired. The ‘fname’ is “Squares.png,” which saves the figure under file name Squares and .png format. The bbox_inches =”tight” save the figure in a tight fit. And pad_inches = 1 gives padding of 1 around the saved figure. Also, the facecolor is ‘g,’ which gives the green color. Finally, the orientation is ‘landscape,’ which saves the figure in landscape mode.
Matplotlib Savefig() formats
1 2 3 4 5 6 7 8 9 10 11 12 13 14 15 16 | import numpy as np import matplotlib.pyplot as plt x = np.linspace(0, 10,20) m = 1 c = 2 y = m*x + c fig = plt.figure() plt.plot(x, y) plt.title("y=mx+c") plt.xlabel('x-axis') plt.ylabel('y-axis') fig.set_size_inches(5, 5) plt.savefig("Figure saved in jpg format.jpg") |
EXPLANATION:
Firstly, to change the figure format using the Matplotlib savefig() function, we need to change the extension of the image file name in the savefig() function. In the above example, the file name’s extension in the savefig() method is .jpg, which saves the figure in jpg format. We can save plots in different formats like .png, .jpg, .pdf, .svg, and many more just by changing the files’ extensions to save as desired in the savefig() methods.
Change size using Matplotlib Savefig() in Python
1 2 3 4 5 6 7 8 9 10 11 12 13 14 15 16 | import numpy as np import matplotlib.pyplot as plt x = np.linspace(0, 10, 0.5) m = 1 c = 2 y = m*x + c plt.figure(figsize=(10, 10)) plt.savefig('750x750.png', dpi=75) plt.plot(x, y) plt.title("y=mx+c") plt.xlabel('x-axis') plt.ylabel('y-axis') plt.show() |
OUTPUT:

EXPLANATION:
To change the figure’s size using savefig(), we need to set the figure dimension in inches while instantiating the figure. And later, the dpi (Resolutions in dots per inch) is specified so that the dimensions of the saved image are correct.
Conclusion
In today’s article, we learned how to save figures with real-time examples using the plt.savefig() function. We used different methods and different combinations of parameters as per our choice. The best coding practice is to try all possible combinations of methods and parameters. Refer to this article for any queries related to the Matplotlib savefig() in Python.
However, if you have any doubts or questions, do let me know in the comment section below. I will try to help you as soon as possible.
Happy Pythoning!